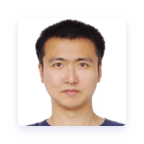
Understanding and Improving Defensive Driving Behaviors in Vehicles with GB/T Level-2 Driving Automation
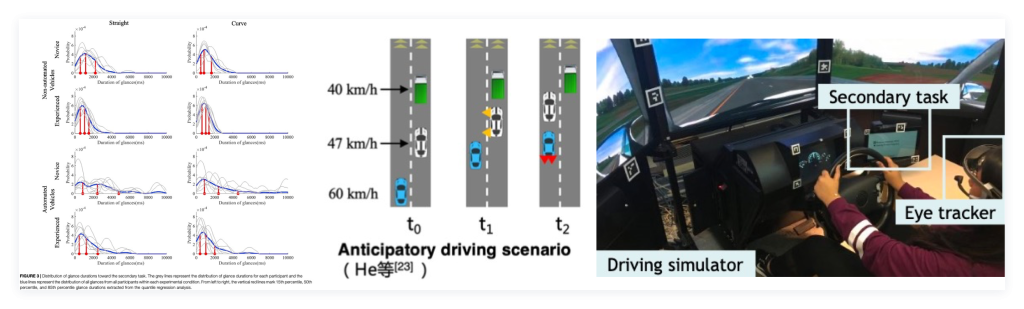
The popularization of driving automation systems in recent years has raised safety concerns on adopting these systems. In the foreseeable future, drivers are still required to monitor the road and the automation, and take over the control of the vehicle when necessary in vehicles with driving automation. Thus, defensive driving, which is critical to driving safety in non-automated vehicles, may still enhance driving safety in vehicles equipped with driving automation. Defensive driving can allow drivers to respond to or get ready to respond to critical situations in advance and increase their time buffer before takeover events. However, very few studies have investigated defensive driving in vehicles with driving automation. How defensive driving can be influenced by drivers’ mental model, hazard perception and other potential factors is still unclear, and very few strategies have been proposed to facilitate drivers’ defensive driving behaviors in vehicles with driving automation. In this project, we aim to introduce the concept of defensive driving into vehicles with GB/T Level-2 driving automation, and investigate the factors that may influence drivers’ defensive driving behaviors through survey studies, driving simulation, video simulation, instrumented vehicle experiments and naturalistic data collection. Then, we plan to propose and evaluate strategies (e.g., in-vehicle display design and driver training program) that can facilitate defensive driving in vehicles with GB/T Level-2 automation, and analyze the mechanisms of these facilitating strategies. The outcome of this project can provide theoretical guidelines and practical experience for the design of behavior intervention strategies for users of driving automation.
Understanding and mitigating distracted driving behaviors among delivery person
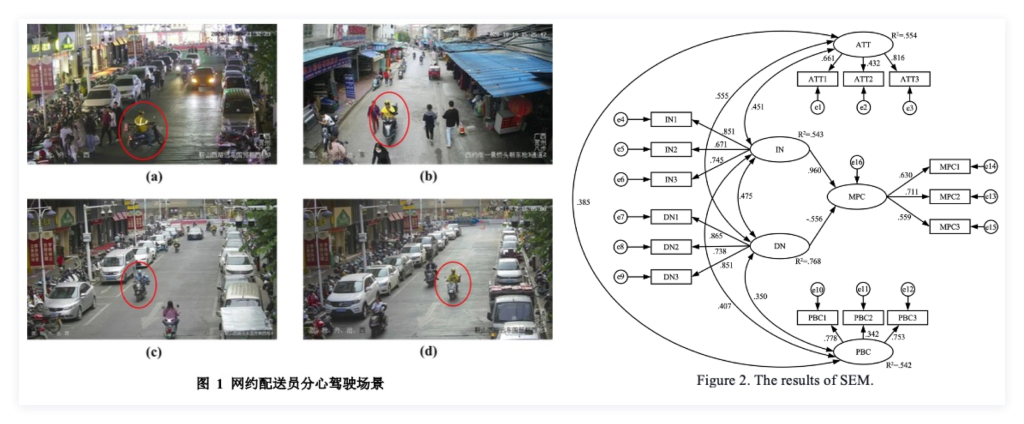
With the rapid development of Online-to-Offline (O2O) marketing in China, online food delivery has had a tremendous and continuous expansion since 2011. In the delivery task, delivery workers usually involve in tasks that are not related to riding (non-riding-related tasks, or NRRT), leading to distracted riding. Delivery workers have to share the road with other road users, including vehicles and pedestrians. In recent years, distracted riding among delivery workers has become a tremendous threat to traffic safety in urban area in China, which, unfortunately, have NOT yet been investigated. This project aims to improve the riding safety of delivery persons by optimization of delivery procedures and interface design of the corresponding smartphone applications, based on the understanding the factors leading to distracted riding. New technologies may also be tested and applied in this project, such as the AR display in helmet.
Alleviating Battery Electric Vehicle Drivers’ Range Anxiety and Eco-Driving Induction
Range anxiety of one of the major obstacles of electric vehicles (EVs) nowadays. The phenomenon of “range anxiety” can lead to irrational charging decisions and reduce the efficiency of the charging network. Thus, it is necessary to understand the factors leading to range anxiety from both spatial and temporal perspectives of view. Through depicting the characteristics of EV users, it is possible to predict the level of range anxiety of users under different conditions and anticipate their charging decisions. Further, we can provide customized feedbacks to EV users and design training programs of them in order to facilitate more rational charging behaviors and more economic driving style, and thus alleviate their range anxiety. The outcome of this research can improve the social acceptance of EVs, optimize the efficiency of charging network and boost the EV market share in cities.
Research on Driver's High Cognitive Load Management in Assisted Driving Based on Driver State Monitoring
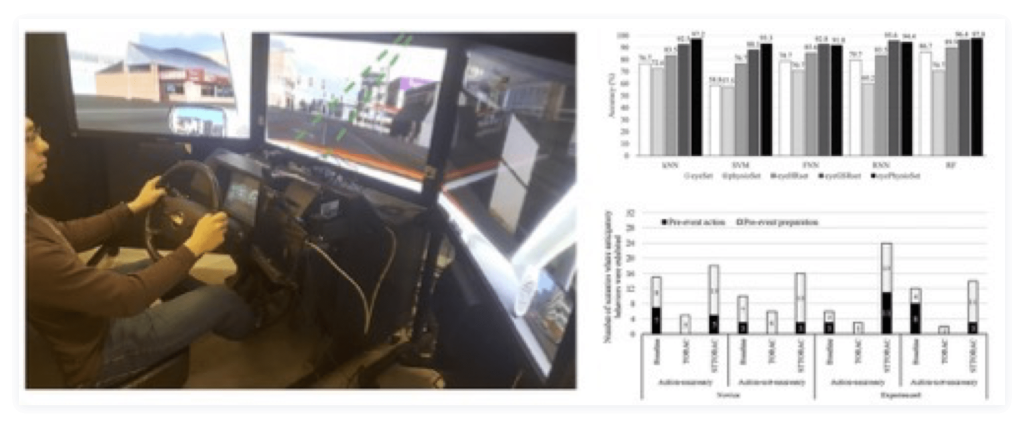
Excessive cognitive load can impede drivers' driving ability, potentially endangering road safety. Even with driving automation (where drivers do not continuously control the vehicle), drivers are still responsible for monitoring road conditions, anticipating potential hazards, and taking over the vehicle when necessary. Consequently, a high cognitive load state in drivers can increase crash risk. However, current studies on driving automation usually neglects the relationship between high cognitive load and driver ability and has not established a threshold for high-risk cognitive load. In addition, existing methods for estimating driver cognitive load rely on research-level equipment, rendering non-inductive monitoring in passenger car cabins difficult. Few studies have focused on alleviation of high cognitive load of drivers in vehicle with driving automation. Therefore, this project aims to explore the relationship between drivers' cognitive load and driving ability when driving automation is controlling the vehicle, develop non-invasive driver states monitoring systems using integrated vehicle sensors and smartwatches, and propose and verify an adaptive method for alleviating high cognitive load (such as in-car interaction design and shared control strategies). The results of this project can serve as a theoretical and practical basis for designing driver states monitoring systems in both non-automated and automated vehicles.
Understanding the interactions between autonomous vehicles and other road users in mixed traffic
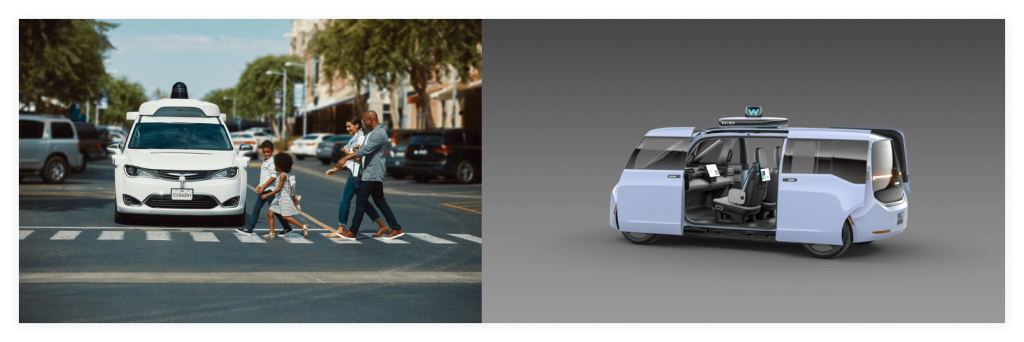
It is often foreseen that in the near future, the traffic flow will be composed of both autonomous vehicles (AVs) and human-driven vehicles (HDVs) while human drivers will remain the majority of operators on the roads. The resulting mixed traffic where AVs and HDVs share the roads with frequent interactions requires detailed modeling of human driving behaviors in highly interactive environments. The research objective is to examine the hypothesis that humans exhibit a different decision-making logic when interacting with AVs in comparison with when interacting with other HDVs in real world. To this end, a mix of statistical and machine learning methods to characterize AV-HDV interactions in various traffic settings, such as car-following and lane-changing. With these findings, this project will lead to the introduction of advanced AV control algorithms that can maintain a desired traffic state by utilizing AVs to influence the behavior of surrounding HDVs based on control theory or reinforcement learning.
On the other hand, although AVs can simulate human drivers in most cases, their behaviors might be different from HDVs, especially in complex traffic situations. In traditional driving tasks, drivers may be able to understand the intention of other road agents; while when sharing the road with AVs, drivers’ capability in anticipating AVs’ behaviors can be affected by multiple factors. Thus, it is necessary to understand the gaming process between AVs and HDVs in mixed traffic and explore external human machine interfaces that can facilitate safer and more efficient interactions between AVs and HDVs. This research will also provide insights on the design of control algorithms and gaming strategies of AVs in complex situations.
Motion Sickness in Vehicles: Understanding and Mitigation
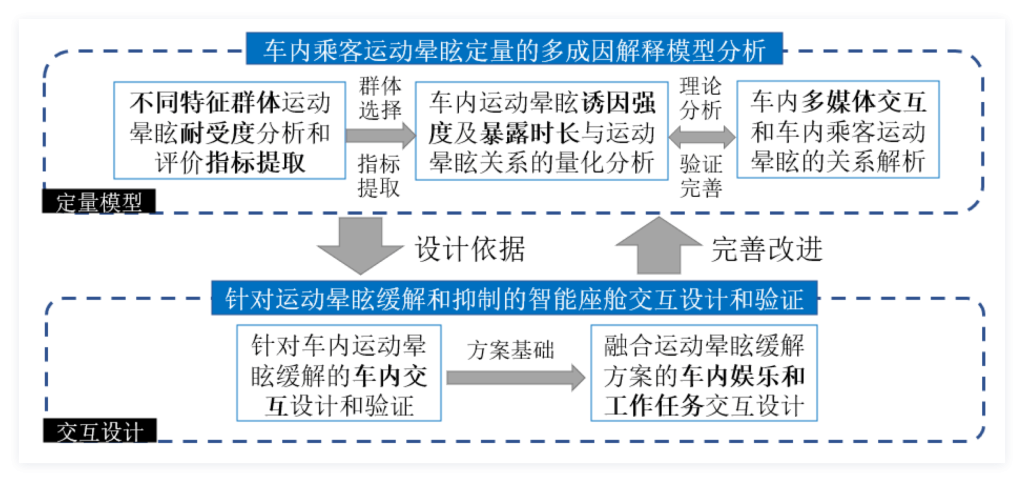
This project will explore the impact of advanced vehicle technologies (new energy, intelligent driving, intelligent cockpit, in-car VR, etc.) on motion sickness among passengers, as well as its causes and solutions. The study plans to quantitatively analyze the causes of motion sickness in passengers after the introduction of advanced vehicle technologies, analyze the weight of different motion sickness inducers in different populations, explore the key threshold of motion sickness inducer intensity and cumulative amount, and establish a multi-factor explanation model for motion sickness. Based on the quantitative analysis of inducers, an adaptive in-car interaction system will be designed to reduce the likelihood of passenger motion sickness during in-car entertainment and work tasks.