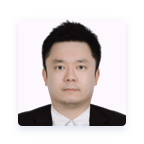
Multi-Vehicle Perception and Semantic Information Fusion Based On Environmental Sensitivity

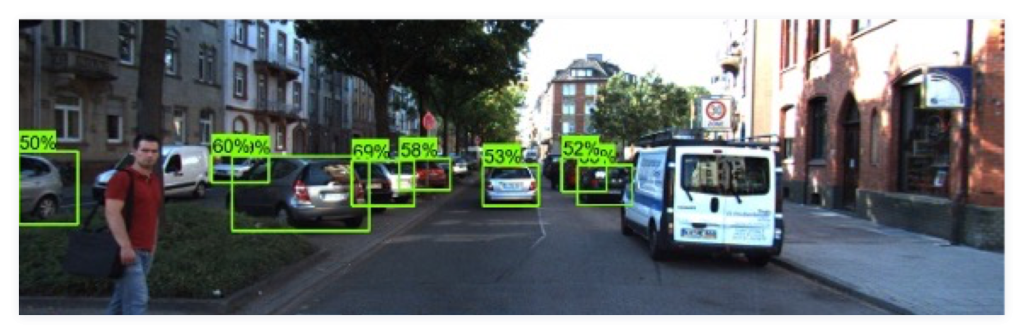
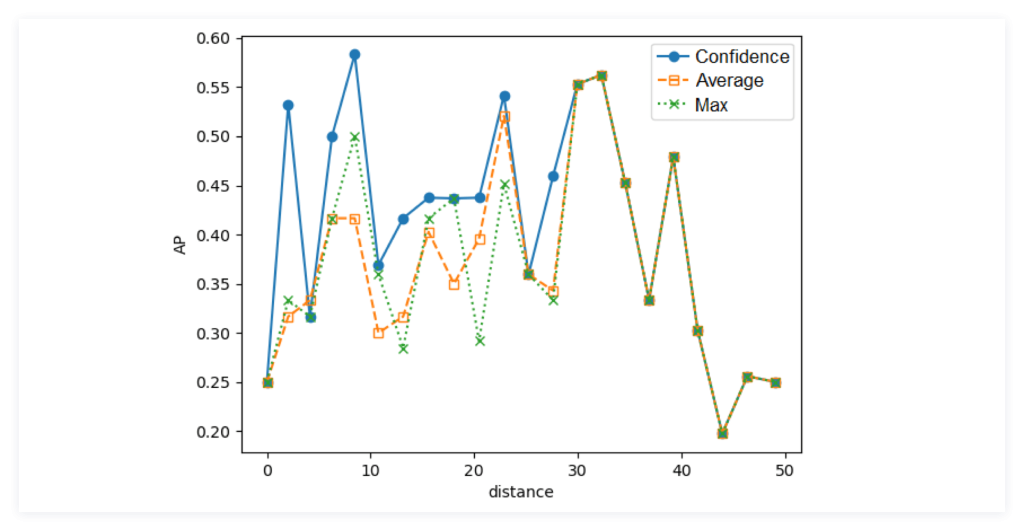
Background:
Autonomous vehicles rely on data collected by sensors and perception algorithms to perceive the surrounding environment, and make driving decisions based on the perception results, and then control the vehicle for actual driving operations according to the decisions. Among them, environmental perception is the most fundamental and critical component of autonomous driving. Based on the data collected by sensors, autonomous vehicles need to infer the real world to reconstruct the scene, extract the semantic information and provide sufficient information for subsequent control operations. However, current autonomous driving technology is still unable to achieve fully autonomous driving and respond promptly to sudden changes in the dynamic environment. The main reason is that the actual driving scenario is much more complex than the existing dataset, and dynamic changes are more frequent. In order to overcome the limitations of single vehicle perception and improve the ability and reliability of autonomous driving vehicles to cope with environmental changes, with the promotion of next-generation wireless communication technology, autonomous driving with connected vehicles has become a new trend in this field of research.
Novelties:
This project will measure the capability of neural networks to perceive environmental changes through an evaluation scheme based on environmental features, targeting different depths of neural networks used in vehicle perception under different environmental conditions. Environmental sensitivity measures the algorithm under different environment. When the algorithm is able to distinguish different types of environmental features and maintain performance in abnormal situations, its results will be more trustworthy. In the context of multi vehicle collaborative perception, in order to trust the perception results from different vehicles, it is urgent to further establish a perception confidence evaluation model based on environmental sensitivity, and use this model to quantify the confidence level of perception result, achieving semantic fusion of multi vehicle target detection in VSN and VCN. Ultimately, mitigating the limitations of single vehicle perception and improving the capability and reliability of autonomous vehicles to cope with dynamic environment.
Project Image 1: Quantification of Environmental Features and Environmental Sensitivity,
Project Image 2: Semantic Objectives and Confidence in Complex Scenarios,
Project Image 3: Semantic Fusion Results Based on Confidence,